
WLHS is verified through numerical examples by comparing the estimated distribution parameters with those from other methods such as Random Sampling and Latin Hypercube Sampling. This paper describes equations and detail procedure necessary to apply weight function to WLHS. This method is called Weighted Latin Hypercube Sampling (WLHS). Latin Hypercube Sampling 100 Monte Carlo Samples 100 Latin Hypercube Samples McKay, Beckman, and Conover, 1979, Technometrics proved that LHSThis paper proposes extension of Latin Hypercube Sampling (LHS) to avoid the necessity of using intervals with the same probability area where intervals with different probability areas are used. Latin Hypercube Designs with Branching and Nested Factors for Initialization of Automatic Algorithm Conguration Simon Wessing simon.wessingtu-dortmund.de Computer Science Department, Technische Universit&228 t Dortmund, Germany Manuel L&243 pez-Ib&225 &241 ez manuel.lopez-ibanezmanchester.ac.uk Alliance Manchester Business School, University of Manchester, UKbut weight them to.
And WLHS proposed in this paper, respectively, are compared to investigate the effect of choosing different sampling techniques.Latin hypercube sampling. In this application, clearance-to-stops (CSs) calculated using LHS proposed by Huang et al. The proposed WLHS is applied to seismically isolated structures in nuclear power plants. Accuracy of WLHS estimation on distribution parameters is depending on the selection of weight function.
Also, in a case where the type of probability distribution of population is known or properly assumable, it is possible to calculate the occurrence probability of subset based on the distribution parameters of population estimated through sampling.All analysis results (e.g., Wedge Weight, Wedge Volume, Normal Force. As the type of probability distribution of population is unknown in most sociology and engineering problems, the expectation of random variable or the occurrence probability of subset can be directly calculated through sampling. In addition to being simple to use, MCS is also the most accurate and computationally expensive of the sampling-based methods.Sampling technique is used to estimate uncertainty inherent in population. Implementation of an MCS needs only a little understanding of probability and statistics.
Nevertheless, in probabilistic design for nuclear power plants associated to ASCE 43 , the probability distribution of outputs produced by the system is properly assumed in order to increase the efficiency in calculation through an allowance for some degree of error. Even if the inputs are fortunately known as normal distribution, the outputs produced by a system may have other types of distribution than normal distribution if the correlation between random variables is nonlinear or the system itself has a nonlinear feature. Carbon in the structure, total dry weight and nitrate concentration was carried.It is often difficult to define the probability distribution of inputs entered in a system to define problems in sociology and engineering. Both random and Latin Hypercube sampling and N 2000 samples were used. Let’s say for example that we. One-dimensional Latin hypercube sampling involves dividing the cumulative density function (cdf) into n equal partitions and then choosing a random data point in each interval.
Latin Hypercube Sampling (LHS) is a method for dividing the whole interval of random variables into several intervals with the same probability area and for selecting samples from each interval. When there is a need to calculate the probability in the tail, Importance Sampling is a method for selecting, randomly in the tail area, a far smaller sample than RS. As Random Sampling (RS) uses samples selected randomly among population, it has a disadvantage of a lengthy calculation time because a large number of samples are required especially in a case where a probability to be calculated is located in the tail of probability distribution.
This method is called Weighted Latin Hypercube Sampling (WLHS). In a case where intervals with different probability areas are used, it is necessary to make a correction using proper weight function. This study proposes extension of LHS to avoid the necessity of using intervals with the same probability area. For this reason, LHS is preferred in probabilistic structural design for an efficient calculation.

Latin Hypercube Sampling With Weight How To Extend LHS
2.1.Latin Hypercube Sampling (LHS)If the number of samples is the same as that of population, an exact probability distribution can be found. Based on this review, weight function and distribution parameters can be defined in a reasonable way. 2.Weighted Latin Hypercube Sampling (WLHS)In order to see how to extend LHS to propose WLHS, it is helpful for us to review on LHS. And WLHS proposed in this paper, respectively, are compared to investigate the effect of choosing different sampling techniques.
However, this method of setting the sample intervals need to have more flexible way because of the following two reasons. The well-known derivation of these equation is shown in Appendix as a reference.(5) 2.2.Weighted Latin Hypercube Sampling (WLHS)In the afore-mentioned Latin Hypercube Sampling, each interval is set so that probability area of each interval is the same, and one sample is selected from each interval. The probability- area center refers to the value of random variables at the center of probability area in each interval.If the population of random variable X follows normal distribution, sample mean X ¯ and unbiased sample variance S ¯ 2 can be estimated as follows, based on samples selected using LHS. In Latin Hypercube Sampling, the total probability area created between probability density function and horizontal axis (random variable axis) is divided into n intervals with the same areas, and n samples are created through a random selection within each interval or a selection of the probability-area center in each interval.
On the other hand, in WLHS method, the probability area in each interval is different from each other. Therefore, the probability of selecting each sample is the same as 1 n. In LHS method, the total probability area is divided into n intervals with the same areas, and n samples are selected from these intervals. These intervals need not have the same probability area, unlike LHS. 2.2.1.Weight function for a single random variableIn WHLS, the total probability area created by probability density function and horizontal axis (random variable axis) is divided into n intervals, and samples are selected from each interval as similarly as in LHS. Second, there is possibility that a sample interval can be optimized to accurately estimate the probability distribution of population using a smaller number of samples than LHS.
In other words, the mean and variance of population with normal distribution (or lognormal distribution) can be approximated more flexibly using sample mean (or median) and sample variance (sample lognormal variance) calculated according to the equations presented in this section. ( 1).Sampling intervals in WLHS can be selected more flexibly than in LHS, in consideration of users’ conveniences or the limits of given data. The weight defined this way is represented by Eq. This sampling method is referred to as Weighted Latin Hypercube Sampling (WLHS). As mentioned above, the probability area (A i) of each interval is the same as probability of selecting a sample from that interval, so that it is used as a weight function.
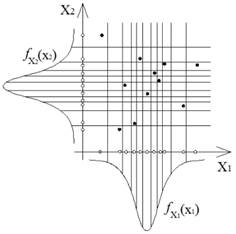
Sample mean and standard deviation are calculated, and their accuracy is verified through their comparison with the known mean and standard deviation of population. Samples are selected from the population using Random Sampling, LHS and WLHS. 1 and 2 show probability density function (PDF) and cumulative distribution function (CDF) of that probability distribution.
In Latin Hypercube Sampling and Weighted Latin Hypercube Sampling, sample intervals are set according to the procedures mentioned in Section 2, and samples are selected at the probability- area center in each interval. In Random Sampling, samples are selected randomly from standard normal distribution. 3.2.Weighted Latin Hypercube SamplingSamples are selected from population using Random Sampling, Latin Hypercube Sampling and Weighted Latin Hypercube Sampling. However, the example having standard normal distribution is considered in this paper.
